Evaluating the Value of the (@)Purge Rule
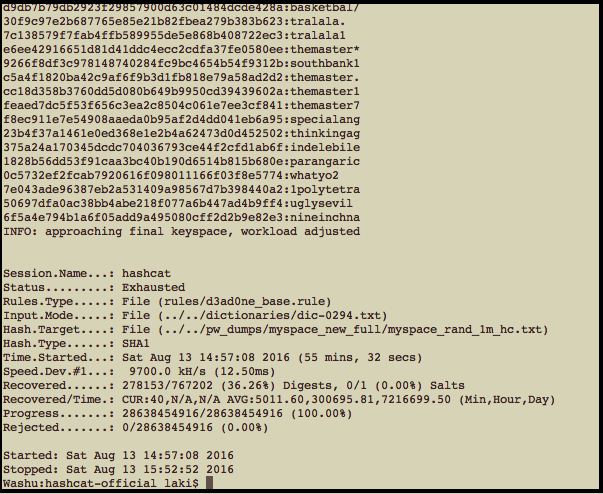
“Only sometimes when we pick and choose among the rules we discover later that we have set aside something precious in the process.” ― Helen Simonson, Major Pettigrew's Last Stand Background and Problem Statement: I was recently asked the following question: "Is there any value in supporting the character purge rule in Hashcat?" The purge rule '@x' will remove all characters of a specific type from a password guess. So for example the rule '@s' would turn 'password' into 'paword'. The full thread can be found on the Hashcat forum here. The reason behind this inquiry was that while the old version of Hashcat implemented the character purge rule, GPU versions of Hashcat and Hashcat 3.0 dropped support for it. Since then, At0m added support for the rule back in the newest build of Hashcat which makes this question much less pressing. That being said, similar questions pop up all the time and I felt it was worth looking into if